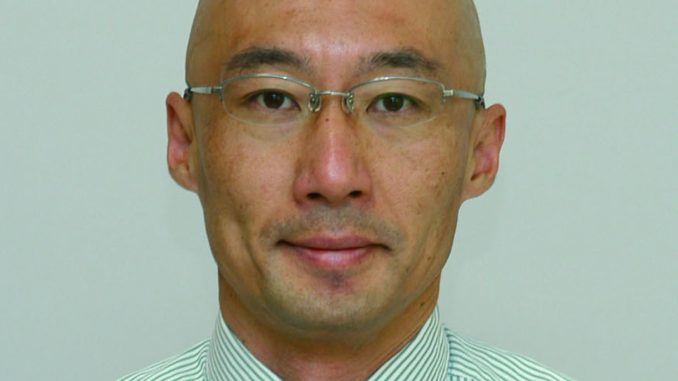
Time: 14:00 on 20/3/2018
Place: room 816, Ta Quang Buu Library, HUST
Presenter: Prof. Hiroshi Mamitsuka, Professor, Kyoto University
Title: Applying “learning to rank” to large-scale MeSH indexing
Abstract:
Learning to rank (LTR) has been developed in information retrieval for ranking documents regarding the relevance to a casted query. Typically LTR builds a ranking model from given relevant (or irrelevant) query-document pairs. I will give a brief summary of LTR, showing the background, focusing on LambdaMART, a LTR software, which used in empirical performance test. Also this survey will show that eventually LTR is an ensemble learning solution for large-scale multi-label classification, where queries are labels. A lot of issues in bioinformatics can be turned into multilabel classification problems having relatively similar properties. One typical example is biomedical document annotation. Currently PubMed, a database of 26 million biomedical citations, has around 30,000 keywords, called MeSH (Medical Subject Headings) terms, i.e. labels in multilabel classification, where the number of articles per MeSH term is extremely diverse, ranging from only 20 to more than eight million. This large, biased dataset already far goes beyond the general sense of regular multilabel classifiers. I will then explain the application of LTR to this huge size of practical multilabel classification in bioinformatics. Finally I will briefly show other possible applications of LTR in bioinformatics. The work in this talk appeared in ISMB in 2015 and 2016.
Prof. Hiroshi Mamitsuka received the B.S. degree in biophysics and biochemistry, the M.E. degree in information engineering, and the Ph.D. degree in information sciences from the University of Tokyo, Tokyo, Japan, in 1988, 1991, and 1999, respectively. He is a professor of Bioinformatics Center, Institute for Chemical Research, Kyoto University, since 2005. Also currently he is a FiDiPro professor of Department of Computer Science, Aalto University, Finland. He has been working on research in machine learning, data mining, and bioinformatics. His current research interests include machine learning on graphs and networks in biology and chemistry.