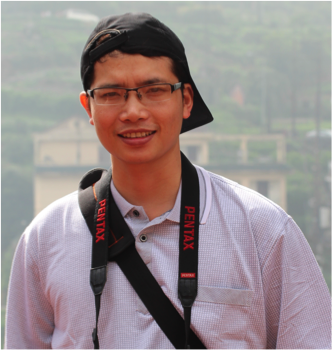
Time: 13:30 on 14/4/2015
Place: KDE Lab, room 1002, building B1, HUST
Presenter: Ngo Van Linh
Title: Effective and Interpretable Document Classification using Distinctly Labeled Dirichlet Process Mixture Models of von Mises-Fisher Distributions
Abstract: Recently, as the number of documents has been rapidly increasing, automatic text categorization is becoming a more important and fundamental task in information retrieval and text mining. Accuracy and interpretability are two important aspects of text classifiers. While the accuracy of a classifier measures the ability to correctly classify unseen data, interpretability is the ability of the classification to be understood by humans and supplies reason why each data instance is assigned to label. This paper proposes an interpretable classification method by using the Dirichlet process mixture (DPM) model to discover the hidden topics distinctly within each label for classification of directional data based on the von Mises-Fisher (vMF) distribution. By using the labeled information of the training data explicitly and determining automatically the number of topics for each label to find the topical space, class topics are coherent, relevant and discriminative and since they help us interpret class’s label as well as distinguish classes. Our experimental results showed the advantages of our approach via significant criteria such as separability,interpretability and effectiveness in classification task of large datasets with high dimension and complex distribution. Our obtained results are highly competitive with state-of-the-art approaches.
Some pics: